Get Started
Tensor
Pytorch is similar with Numpy, but tensor can be accelerated on GPU.
import torch
import numpy as np
numpy_tensor = np.random.randn(2,3)
# Numpy ndarry -> PyTorch Tensor
pytorch_tensor1 = np.Tensor(numpy_tensor)
pytorch_tensor2 = np.from_numpy(numpy_tensor)
# get the shape of the tensor
pytorch_tensor1.shape
pytorch_tensor1.size()
# get the datatype of the tensor
pytorch_tensor1.type()
# get the dimension of the tensor
pytorch_tensor1.dim()
# get the number of all elements in the tensor
pytorch_tensor1.numel()
# create a matrix, which elements are 1 and size is (2,3)
x = torch.ones(2,3)
# create a matrix with random value
x = torch.randn(4,3)
# get the largest value in each row
max_value, max_idx = torch.max(x, dim=1)
# get sum for each row
sum_x = torch.sum(x,dim=1)
Variable
Variable is encapsulation of tensor. There’re three attributes of Variable:.data
.grad
.grad_fn
.
from torch.autograd import Variable
x_tensor = torch.randn(10,5)
y_tensor = torch.randn(10,5)
# tensor -> Variable
x = Variable(x_tensor, requires_grad = True) # require computing gradient
y = Variable(y_tensor, requires_grad = True)
z = torch.sum(x+y)
print(z.data)
print(z.grad_fn)
z.backward()
print(x.grad)
print(y.grad)
Automatically Derivation
import torch
from torch.autograd import Variable
x = Variable(torch.Tensor([2]),requires_grad=True)
y = x + 2
z = y**2 +3
z.backward()
print(x.grad)
x = Variable(torch.randn(10,20), requires_grad=True)
y = Variable(torch.randn(10,5), requires_grad=True)
w = Variable(torch.randn(20,5), requires_grad=True)
# torch.matmul is matrix multiplication
# torch.mean is to get the avgerage value
out = torch.mean(y - torch.matmul(x,w))
out.backward()
Linear Modle & Gradient descent
To the opposite direction of the gradient, we can get the minimum point by updating the value of w and b, till the best w and b with the minimum loss.
Learning rate means “stride”. A large learning rate may causes convergence hardly. A small learning rate may causes waste of time.
import torch
import numpy as np
from torch.autograd import Variable
import matplotlib.pyplot as plt
x_train = np.array([[3.3], [4.4], [5.5], [6.71], [6.93], [4.168],
[9.779], [6.182], [7.59], [2.167], [7.042],
[10.791], [5.313], [7.997], [3.1]], dtype=np.float32)
y_train = np.array([[1.7], [2.76], [2.09], [3.19], [1.694], [1.573],
[3.366], [2.596], [2.53], [1.221], [2.827],
[3.465], [1.65], [2.904], [1.3]], dtype=np.float32)
plt.plot(x_train, y_train,'bo')
x_train = torch.Tensor(x_train)
y_train = torch.Tensor(y_train)
w = Variable(torch.randn(1), requires_grad=True)
b = Variable(torch.randn(1), requires_grad=True)
x_train = Variable(x_train)
y_train = Variable(y_train)
def linear_modedl(x):
return x * w + b
y_ = linear_model(x_train)
plt.plot(x_train.data.numpy(), y_train.data.numpy(), 'bo', label='real')
plt.plot(x_train.data.numpy(), y_.data.numpy(), 'ro', label='estimated')
plt.legend()
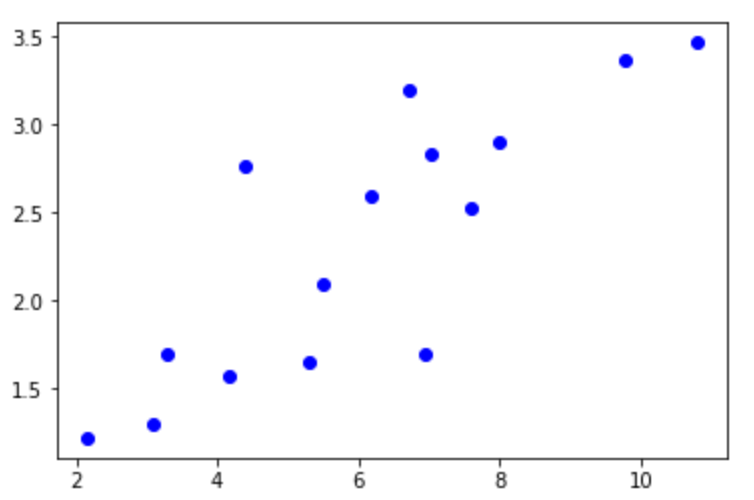
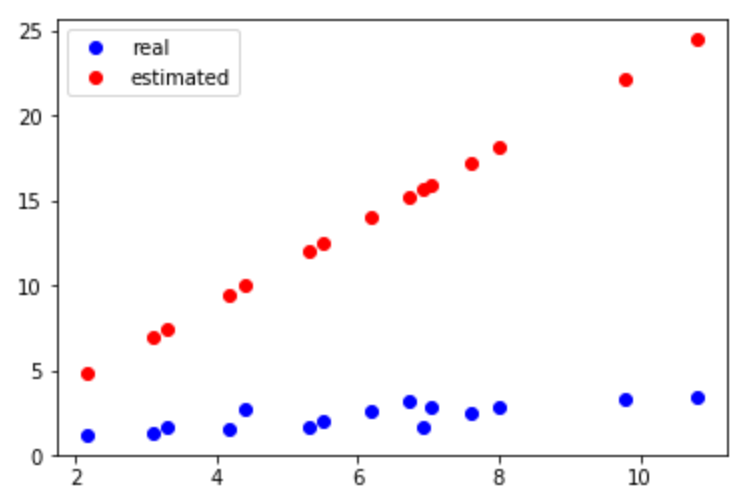
The left img shows the original data. The right img shows the result that only updates w and b by one time.
def get_loss(y_, y_train):
return torch.mean((y_ - y_train) ** 2)
loss = get_loss(y_, y_train)
loss.backward()
w.data = w.data - 1e-2 * w.grad.data
b.data = b.data - 1e-2 * b.grad.data
for i in range(10):
y_ = linear_model(x_train)
loss = get_loss(y_,y_train)
w.grad.zero_()
b.grad.zero_()
loss.backward()
w.data = w.data - 1e-2 * w.grad.data
b.data = b.data - 1e-2 * b.grad.data
print('epoch: {}, loss: {}'.format(i, loss.data[0]))
y_ = linear_model(x_train)
plt.plot(x_train.data.numpy(), y_train.data.numpy(), 'bo', label='real')
plt.plot(x_train.data.numpy(), y_.data.numpy(), 'ro', label='estimated')
plt.legend()
plt.show()
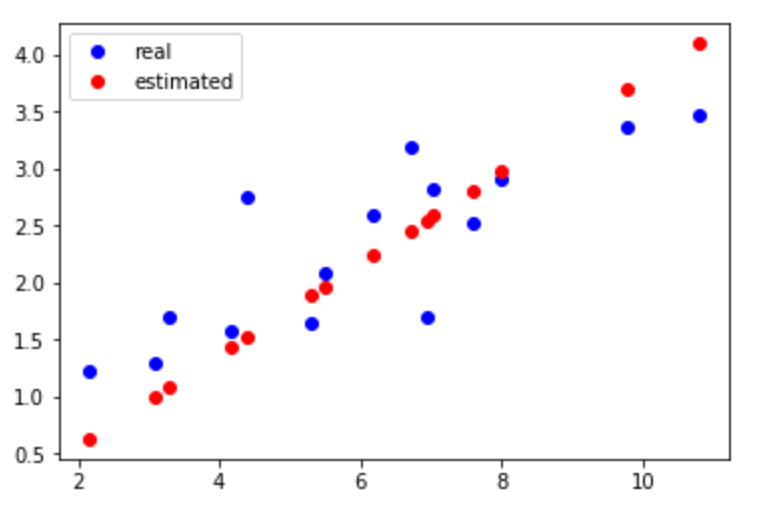
We can see the final result completes linear regression.
Below is something about plt()
Various line types, plot symbols and colors may be obtained with
plot(X,Y,S) where S is a character string made from one element
from any or all the following 3 columns:
b blue . point - solid
g green o circle : dotted
r red x x-mark -. dashdot
c cyan + plus -- dashed
m magenta * star (none) no line
y yellow s square
k black d diamond
w white v triangle (down)
^ triangle (up)
< triangle (left)
> triangle (right)
p pentagram
h hexagram
Initial Parameters
import numpy as np
import torch
from torch import nn
class sim_net(nn.Module):
def __init__(self):
super(sim_net, self).__init__()
self.l1 = nn.Sequential(
nn.Linear(30, 40),
nn.ReLU()
)
self.l1[0].weight.data = torch.randn(40, 30) # initial for one layer
self.l2 = nn.Sequential(
nn.Linear(40, 50),
nn.ReLU()
)
self.l3 = nn.Sequential(
nn.Linear(50, 10),
nn.ReLU()
)
def forward(self, x):
x = self.l1(x)
x =self.l2(x)
x = self.l3(x)
return x
for i in net2.children():
print(i)
for i i in net2.modules():
print(i)
for layer in net2.modules():
if isinstance(layer, nn.Linear):
param_shape = layer.weight.shape
layer.weight.data = torch.from_numpy(np.random.normal(0, 0.5, size=param_shape))
torch.nn.init
from torch.nn import init
print(net2[0].weight)
init.wavier_uniform(net2[0].weight)
Batch/Dataloader
Dataloader
is the tool to package data, firstly we should convert data from numpy array or other format to Tensor, and then put it in the Dataloader
. It can help us iterate data efficiently.
MNIST
Image.shape = [1,28,28]
import os
import torch
import torch.nn as nn
from torch.autograd import Variable
import torch.utils.data as Data
import torchvision
import matplotlib.pyplot as plt
import numpy as np
torch.manual_seed(1)
EPOCH = 5
BATCH_SIZE = 50
LR = 0.001
DOWNLOAD_MNIST = False
train_data = torchvision.datasets.MNIST(
root = './mnist',
train=True,
transform = torchvision.transforms.ToTensor(),
download = DOWNLOAD_MNIST,
)
test_data = torchvision.datasets.MNIST(root = './mnist', train=False)
train_loader = Data.DataLoader(dataset = train_data, batch_size=BATCH_SIZE, shuffle=True)
test_x = Variable(torch.unsqueeze(test_data.test_data, dim=1), volatile=True).type(torch.FloatTensor)[:2000]/255. # shape from (2000, 28, 28) to (2000, 1, 28, 28), value in range(0,1)
test_y = test_data.test_labels[:2000]
class CNN(nn.Module):
def __init__(self):
super(CNN, self).__init__()
self.conv1 = nn.Sequential(
nn.Conv2d(
in_channels=1,
out_channels=16,
kernel_size=5,
stride=1,
padding=2,
),
nn.ReLU(),
nn.MaxPool2d(2),
)
self.conv2 = nn.Sequential(
nn.Conv2d(16,32,5,1,2),
nn.ReLU(),
nn.MaxPool2d(2),
)
self.out = nn.Linear(32*7*7, 10)
def forward(self,x):
x = self.conv1(x)
x = self.conv2(x)
x = x.view(x.size(0),-1)
output = self.out(x)
return output
cnn = CNN()
optimizer = torch.optim.Adam(cnn.parameters(),lr = LR)
loss_func = nn.CrossEntropyLoss()
losses = []
acces = []
for epoch in range(EPOCH):
train_loss = 0
train_acc = 0
for count,(x,y) in enumerate(train_loader):
b_x = Variable(x)
b_y = Variable(y)
output = cnn(b_x)
loss = loss_func(output, b_y)
optimizer.zero_grad()
loss.backward()
optimizer.step()
train_loss += loss.data[0]
pred = torch.max(output,1)[1]
num_correct = (pred == b_y).sum().data[0]
acc = num_correct/b_x.shape[0]
train_acc += acc
losses.append(train_loss/len(train_loader))
acces.append(train_acc/len(train_loader))
print('EPOCH:',epoch,',train loss:',train_loss/len(train_loader),',train acc:',train_acc/len(train_loader))
plt.title('train acc')
plt.plot(np.arange(len(acces)), acces)
plt.show()
CIFAR10
import numpy as np
import torch
from torch import nn
from torch.autograd import Variable
from torchvision.datasets import CIFAR10
torch.manual_seed(1)
def data_tf(x):
x = np.array(x, dtype='float32') / 255
x = (x - 0.5) / 0.5
x = x.transpose((2, 0, 1))
x = torch.from_numpy(x)
return x
train_set = CIFAR10('./data', train=True, transform=data_tf)
train_data = torch.utils.data.DataLoader(train_set, batch_size=64, shuffle=True)
test_set = CIFAR10('./data', train=False, transform=data_tf)
test_data = torch.utils.data.DataLoader(test_set, batch_size=128, shuffle=False)
class VGG(nn.Module):
def __init__(self):
super(VGG,self).__init__()
self.conv1 = nn.Sequential(
nn.Conv2d(
in_channels=3,
out_channels=64,
kernel_size=3,
stride=1,
padding=1,
),
nn.ReLU(),
nn.MaxPool2d(kernel_size=2)
)
self.conv2 = nn.Sequential(
nn.Conv2d(64, 128, 3, 1, 1),
nn.ReLU(),
nn.MaxPool2d(2)
)
self.conv3 = nn.Sequential(
nn.Conv2d(128, 256, 3, 1, 1),
nn.ReLU(),
nn.Conv2d(256, 256, 3, 1, 1),
nn.ReLU(),
nn.MaxPool2d(2)
)
self.conv4 = nn.Sequential(
nn.Conv2d(256, 512, 3, 1, 1),
nn.ReLU(),
nn.Conv2d(512, 512, 3, 1, 1),
nn.ReLU(),
nn.MaxPool2d(2)
)
self.conv5 = nn.Sequential(
nn.Conv2d(512, 512, 3, 1, 1),
nn.ReLU(),
nn.Conv2d(512, 512, 3, 1, 1),
nn.ReLU(),
nn.MaxPool2d(2)
)
self.fc = nn.Sequential(
nn.Linear(512, 100),
nn.ReLU(),
nn.Linear(100, 10)
)
def forward(self, x):
x = self.conv1(x)
x = self.conv2(x)
x = self.conv3(x)
x = self.conv4(x)
x = self.conv5(x)
x = x.view(x.shape[0], -1)
x = self.fc(x)
return x
net = VGG()
optimizer = torch.optim.SGD(net.parameters(), lr=1e-1)
criterion = nn.CrossEntropyLoss()
def train(net, train_data, valid_data, num_epochs, optimizer, criterion):
for epoch in range(num_epochs):
train_loss = 0
train_acc = 0
for im, label in train_data:
im = Variable(im)
label = Variable(label)
output = net(im)
loss = criterion(output, label)
optimizer.zero_grad()
loss.backward()
optimizer.step()
train_loss += loss.data[0]
pred = torch.max(output,1)[1]
num_correct = (pred == label).sum().data[0]
acc = num_correct/im.shape[0]
train_acc += acc
# train_acc += get_acc(output, label)
print('EPOCH:',epoch,',train loss:',train_loss/len(train_data),',train acc',train_acc/len(train_data))
train(net,train_data,test_data,10,optimizer,criterion)